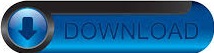

Drawing upon the Helping Theory, we investigate three enablers of volunteering applied to LDW maintenance: impetus to respond, positive evaluation of contributing and increasing awareness. Promoting consumption, adaptation and reuse might all help to increase the user base, and in so doing, might provide the critical mass of volunteers, current LDW projects lack. By making LDWs open, we permit others not only to inspect (hence, increasing trust and consumption), but also to maintain (to cope with API upgrades) and reuse (to adapt for their own purposes). The strategy: becoming open source and turning towards dedicated platforms.

Other projects in the past faced similar issues. Hence, for API-based LDWs to become a sustainable foundation for the Web of Data, we should recognize LDW maintenance as a continuous effort that outlives their breakout projects. Unfortunately, LDWs are fragile upon upgrades on the underlying APIs, compromising LDW stability. Linked Data Wrappers (LDWs) turn Web APIs into RDF end-points, leveraging the Linked Open Data cloud with current data. The review highlights the tools, methods, types of data sources, ontologies, and publication methods, together with the challenges, limitations, and lessons learned in the knowledge graph creation processes. The review takes into account four prominent publication venues, namely, Extended Semantic Web Conference, International Semantic Web Conference, Journal of Web Semantics, and Semantic Web Journal. In this paper, we provide a systematic literature review on knowledge graph creation from structured and semi-structured data sources using Semantic Web technologies. Particularly, linked data and ontologies have been quite essential for data sharing, discovery, integration, and reuse. One of the central concepts in this area is the Semantic Web, with the vision of providing a well-defined meaning to information and services on the Web through a set of standards. Knowledge graphs have, for the past decade, been a hot topic both in public and private domains, typically used for large-scale integration and analysis of data using graph-based data models.
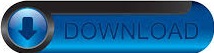